Research In Our Group
Artificial Intelligence
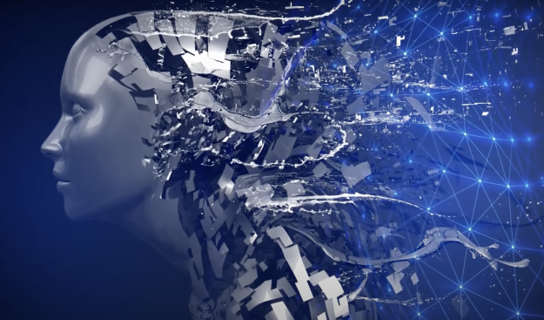
Artificial Intelligence (AI) is quickly becoming the single most essential toolbox in almost every data-intensive field. In fundamental experimental sciences like Physics, this is especially true. Will AI allow us to ask and answer new questions not previously dreamed of, or will we continue to be part of the exploration at all? It's been suggested that the accelerated advancement of AI will quickly change the landscape of many fields of study in medicine, businesses, law, economics, education, and more, vastly changing the direction of potential careers in essentially all fields. Does it still make sense to pursue science? The advancement of AI may eliminate the need for many professions. Will the inevitable continuous advancement in AI replace the need for scientists?
No, probably not. It's more likely that, like with many other fields, science will change and the scientist will change to accommodate. The methods of performing experiments will change to collect and process data with optimization outside of the scope achievable by any previous means. Theoretical physics will also change, with less emphasis on pen and paper theory yielding to leadership from machine modeling. As general-purpose AI evolves theoretical physics will quickly change with generative modeling and simulations guiding most of the experimental efforts. This doesn't mean that there will be no theoretical physicist. At least not right away. But it does mean that the job description is likely going to be changing very fast.
The tools and techniques in modern-day machine learning and AI truly represent a fundamentally new way of doing science. Generative modeling can deduce from an extensive array of possible theoretical frameworks the most probably solution space for a given set of data and suggest the optimal set of needed experimental data to make further constraints based solely on the information within the data. This can be done without any prior or programmed knowledge of what physical processes might be at work.
In Hight Energy, Nuclear, and Particle Physics and particularly Spin Physics or any field in physics where the degrees of freedom are quantum mechanical in nature, theory, and modeling is particularly challenging. Quantum chromodynamics (QCD) as an example is the theory of the strong force and is a beautiful example of how the physical laws that describe a vast degree of quantum complexity can be represented in a single elegant mathematical statement. However, even after 50 years, the theory has yet to be experimentally verified and much of the time experiments meant to deliver this type of verification end up leading to more unanswered questions. This is not because QCD is wrong, it's just incredibly hard to solve the fundamental equations that are required to provide practical information about observables that can be tested. At higher energies where the strong force is less dominant, perturbation theory (pQCD) is helpful where each term in the expansion demands more sophisticated numerical methods of computation. For scales where the strong force is still dominant, which is true for almost all baryonic matter around us, approximate solutions can only be made with large-scale computing using lattice QCD (LQCD). For an understanding of complex composite quantum systems on the partonic level, like in Spin Physics, very little can be definitively said at this stage. This is why phenomenology is at present still an active field. Most of the time these models can provide some direction and motivation to the experimental effort. But generally, only a loose connection to QCD can be made, provided there is enough experimental information obtainable to constrain the parameter space. AI will have an enormous impact here by processing both the information and limitations in the pQCD and LQCD calculations that are presently achievable and the source and error of that information rendering hyper tuned generative models that are self-improving no doubt provide breakthrough insights based on the integration of what we know about QCD, what experimental information we have, and what experimental information we still need to collect.
AI's role in physics will continue to grow and yes both experimental and theoretical careers will change as a result. And it's true that on a long enough time scale there is no dimension of either theoretical or experimental research that is done today that AI and automation can not do far better. But that is only the research that is done today. The field is continuing to evolve, as are we.